Special Section sponsored by
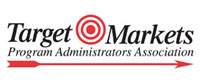
Milliman Executive Sees Broader Data Management Options for Smaller Program Administrators
The insurance industry is like many other industry sectors; in many cases, they
suffer from information overload. For many organizations outside the insurance
business, it’s a situation that appears to be getting worse every year. However, unlike most
other business sectors, the data that is collected within the insurance
industry is quite valuable. While data has always been at the core of the
insurance industry for tasks such as pricing, reserving, etc., today even more
value can be extracted from it.
Quality data has always been a critical requirement for meaningful forecasting
within the insurance industry. However, over the past 10 years, thanks in large
part to the advances in technology, data mining, and modern statistical
methods, a concept known as predictive analysis has become an important tool for successful insurers. While predictive analysis
has been in use within the insurance industry for well over a decade, recent
enhancements to the process now allow it to be used on smaller books of business, and thus it can offer progressive program managers a
competitive advantage for their larger programs.
Predictive analysis basics
One of the organizations that has led the advancements in predictive analysis
has been the actuarial firm of Milliman, Inc. According to Bill Carpenter a
principal and consulting actuary with Milliman, predictive analysis can be
defined as a “process of finding relationships between past data and outcomes that can be used
to predict future outcomes.”
Further, he notes, “Outcomes can be any ‘business result’ that you want to impact,” everything from loss ratios to retention rates to acquisition costs, to name a
few. This flexibility has proven to be highly beneficial in building strategies
to maximize the profitability and growth of a program.
An obvious example of predictive analysis is the credit scoring used by the
three major credit bureaus. In fact, Carpenter states, “It was the banking and credit industry that were among the first to use
predictive analysis over 40 years ago.” He also notes that use by the insurance industry has grown more recently, since
it has become significantly easier to identify trends, patterns and specific
relationships.
But, he says, “Initially, the use of predictive analysis was limited to only large insurers
like Progressive and Allstate.” This was primarily because it would take a firm this large to provide a data
base and the computing power sufficient for the analysis.
However, Carpenter points out that recently the tools and software available to
actuaries have been greatly improved. “The new software is much more forgiving with regard to flaws in the data,” he says. “In the past this would have been a major roadblock to predictive analysis usage.
It was not uncommon for a company to take a year or longer just to clean up the
data and make it useful.”
Carpenter notes that compared to previous predictive analysis tools, today’s software is “much more developed for finding relationships in the data, without having to
tell the software where to look.” All this makes predictive analysis easier to use and thus more appropriate for
smaller books of business, Carpenter points out. The ability to use this
process on smaller books can help program administrators identify their most
profitable customer segments. He points out that this can frequently lead to
revisions in marketing and pricing strategies, or modifications of the
underwriting criteria.
Advancement in the process of predictive analysis has made it “more viable for use with smaller books of business and still come out on the
positive side of a cost/benefit analysis on the process,” according to Carpenter. Today, he says, this can work with programs in the $25
million to $35 million premium range. It’s important to realize the competitive advantages that can be derived from
predictive analysis.
“This puts the whole negotiation process in a different light.” For example, program managers that utilize predictive analysis can identify
their better business and develop strategic approaches to take advantage of the
insights gained about the program, says Carpenter. And he says, “It can also allow for more favorable negotiations with current carriers,
producers or even prospective insurers.”
Predictive analysis can provide a better opportunity for program managers to
utilize the key data from their programs. As a result, predictive analysis has
rapidly become an insurance industry best practice application. Carpenter
indicates that insurance organizations “can no longer look at data as just a cost of doing business.” Rather, “those organizations that consider data as an asset are frequently the most
successful.”
He goes on to say that in today’s hyper-competitive market, program managers that wish to differentiate
themselves from the crowd “should seriously consider predictive analysis for their books of business.” Currently, some program administrators are now realizing what a number of
insurance companies found out years ago: “Their data can be a key source of strategic differentiation,” Carpenter says.
|