Catastrophe modeling
Anything but an exact science
By Joseph J. Hunter-Lattak, CPCU
The American Association of Insurance Services (AAIS) annual conference held in April included a discussion of catastrophe modeling by Robert Healy, senior vice president of ABS Consulting. Catastrophe modeling, commonly referred to as cat modeling, is a risk management tool that uses computer technology to calculate potential losses caused by natural and, in some cases, man-made catastrophes.
Natural catastrophes include hurricanes, earthquakes, tornados, flood, windstorm, hail and wildfires. Some hurricane models include storm surge and inland flooding. Some earthquake models include fire following the event and damage from sprinkler leakage. Man-made catastrophes include acts of terrorism and war.
Cat models contribute to quantifying the potential financial impact of these events. As such, cat modeling is helpful to insurers, reinsurers, businesses and government agencies to measure the total impact of such events and in large measure represents the point where the disciplines of statistics, mathematics, actuarial, geology, seismology, meteorology and engineering meet.
The history of cat modeling
Cat modeling got its start in the 1980s and became much more viable and accepted as computer capability and function became more sophisticated. It really took off following Hurricane Andrew in 1992 and the Northridge earthquake in 1994, as insurance companies scrambled to evaluate, underwrite and price for these and similar natural catastrophes. At the present time, some ratings agencies consider catastrophic loss the primary threat to the property/casualty insurance industry’s financial strength. In some cases, consumer reactions disputing the results and findings and advocating greater understanding of what goes into the models has led to increased scrutiny of catastrophe modeling by insurance regulators. However, the record dollar value and number of claims from the hurricane activity in 2004 and 2005 clearly illustrate that cat models alone cannot accurately predict risk.
Flaws of cat models
Cat modeling is afflicted by issues involving low frequency and high severity, insufficient numbers of cases and claims data, insufficient experience, factors simply not suited to traditional actuarial techniques and the inclusion of random variables. All models include some “biases,” which are the result of simplifying and reducing the number of factors involved and, essentially, “cutting corners.” In addition, they are all dynamic, non-linear and have many “moving parts.” Despite the daunting nature of these difficulties, the one thing to keep in mind, according to Healy, is that “There are not enough exposures in an underwriter’s life to replicate the predictive power of modeling.”
Cat models attempt to be accurate, but they are not precise. A 10% variance is not bad at all, and a standard deviation of one-half to one is about the right level. And assumptions abound! As Healy points out, these are probabilistic tools. Garbage in—garbage out applies, since poor data quality has consequences. In addition, some components of loss, such as flood and storm surge in the case of Hurricane Katrina, varying claims-settling practices among insurance companies and the influence of politics on claim settlements are not factored into most models.
What goes into a typical model?
Natural cat models include many of the things that readily come to mind, as well as a few that don’t. The first is the hazard component, which is simply the event being modeled. For example, the hurricane hazard includes the projected path, wind speed, angles and landfall locations. Next is the inventory portion. This is information on the properties being analyzed, also referred to as exposure data. This includes geocoding data, such as street address, postal code or county; and physical characteristics, such as construction, occupancy, age or height. These two components analyze the physical impact of the catastrophe event. Based on this information, the dollar value of loss to the insurer’s portfolio is then evaluated, based on the insurance provided, limits, valuation and deductibles. Cat models may also include indirect losses, such as the impact of loss of business income and allocated loss adjustment expenses (ALAE), in addition to direct physical losses.
Some models take into account policy and financial data from insurers and reinsurers, such as total values, limits, deductibles and attachment points where reinsurance begins to apply, as well as historical disaster and scientific information to create a probability of loss profile from different events at differing levels. This provides the means to correlate expected losses from a single event or multiple events that affect more than one territory. The number of factors that can be included must be considered and limited when determining the results desired, and this is where biases and “cutting corners” comes into play.
The end product and who uses it
The output from the cat model represents estimates of the losses that the model predicts will occur from a specific event or a set of events. Probabilistic model output is either a probabilistic loss distribution or a set of events that could be used to create a loss distribution. Probable maximum losses (PMLs) and average annual losses (AALs) are calculated from the loss distribution. With these model outputs, underwriters can better analyze and evaluate the overall amount of capital required to pay potential claims and allocate funds between individual risks within that portfolio. This can serve to guide underwriting strategy and the type and amount of reinsurance to purchase.
Insurance departments in some states allow insurers to use cat models in their rate filings to determine premium charges for their customers in catastrophe-prone areas. Insurance rating agencies use them to assess the financial strength of insurers that take on catastrophe risk exposures. The reinsurance industry uses them to assist in structuring and pricing reinsurance treaties. Cat bond investors, investment banks and bond rating agencies use cat models to price and structure catastrophe bonds.
And some models can be adjusted to include the option of including demand surge, or post-event inflation, in the loss estimates. For example, after a disaster event, construction material and labor are usually in short supply initially and this can cause construction costs to be inflated.
Shortcomings of models
After the unprecedented storm frequency and severity of the 2004 and 2005 hurricane seasons, catastrophe modeling faced much criticism for the underestimation of losses. Catastrophe models, like financial models, offer no guarantees. They are only as good as the data fed into them at a certain point in time and the way they are interpreted by the parties that use them. They are also dynamic—constantly being reassessed and updated with new data inputs to reflect changing loss experience and increases in projected losses. For example, the cat models that applied to Hurricane Katrina were inadequate. Several factors, such as unexpected storm surges and the resulting flooding, affected their accuracy. The cat modelers used the lessons learned from their mistakes to refine their programs to account for the powerful storm activity expected in the coming years, some of which is being attributed to global warming. Katrina showed that relying on cat models exclusively can have disastrous results, but it also showed that including such models in the underwriting discipline will ultimately benefit insurance companies and consumers alike.
Conclusion
Catastrophe models produce different results, depending on data inputs, demands and expectations of users, geographic, meteorological and historic data and probabilities relating to insured events and potential losses. Hazard and damage uncertainty and variability issues continue to confound many models. Damage correlation also varies, depending on building codes and practices. Near-term projections vary significantly from long-term projections. Models differ based on their individual behaviors and biases. For this reason, multiple model outputs should be used to understand risk and uncertainty. Healy captured the essence of the issue quite succinctly when he concluded; “There are so many moving parts in the models. Just changing a few assumptions can lead to different results.” *
|
|
|
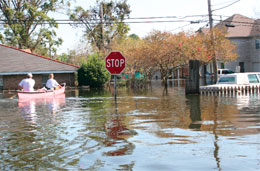 |
|
Catastrophe models produce different results, depending on data inputs, demands and expectations of users, geographic, meteorological and historic data and probabilities relating to insured events and potential losses. |
|